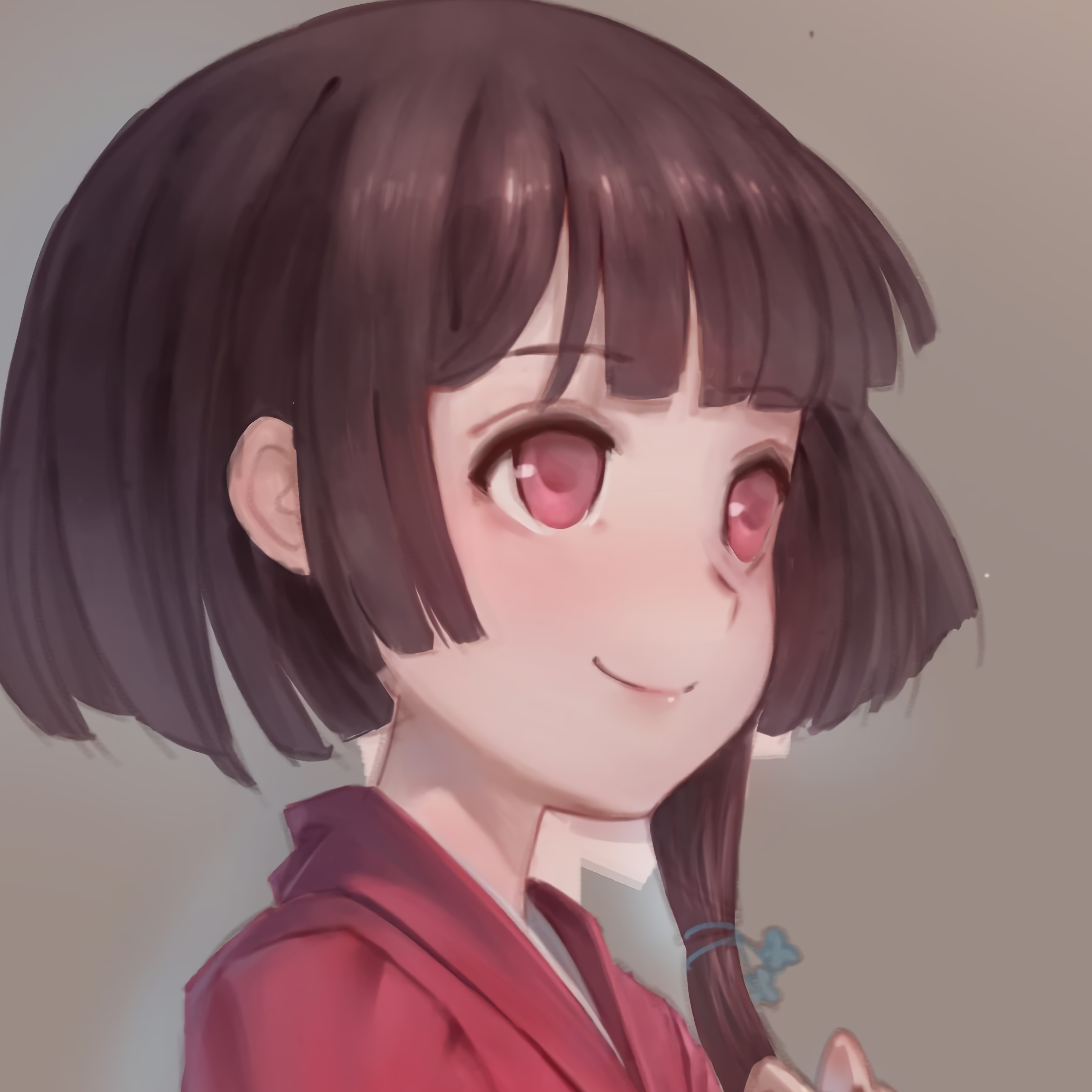
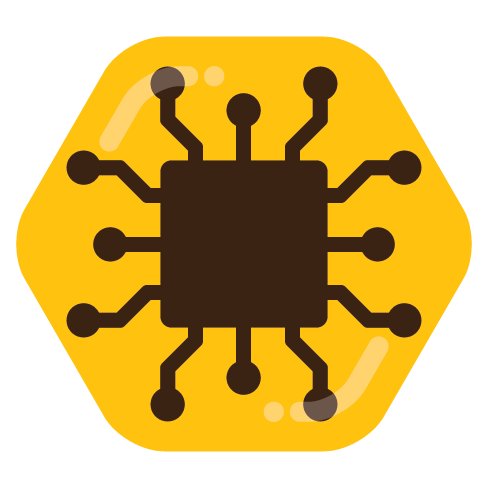
But we could have an average of 684 9/11’s a hour, the actual number of car crashes per hour in the US, driven purely by the piloting skills of the average Amarican driver given command of an aircraft, who wouldn’t want to live in that future?
But we could have an average of 684 9/11’s a hour, the actual number of car crashes per hour in the US, driven purely by the piloting skills of the average Amarican driver given command of an aircraft, who wouldn’t want to live in that future?
“A computer can never be held accountable, therefore a computer must never make a management decision.”
Even more importantly when it comes to assessing properly, machine learning, now referred to as AI, has been continuealy shown to not just repeat the biases in its training data, but to significantly exaggerate them.
Given how significantly and explicitly race has been used to determine and guide so much property and neighborhood development in the training data, I do not look forward to seeing a system that is not only more racist than a post war city council choosing where to build new moterways but which is sold and treated as infallible by the humans operating and litigating it.
Given the deaths and disaster created by the Horizon Post Office Scandel, I also very much do not look forward to the widespread adoption of software which is inherently and provablly far less accurate, reliable, and auditable than the Horizon software. At least that could only ruin your life if you were a Postmaster and not just any member of the general public who isn’t rich enough to have your affairs handled by a human.
But hey, on the bright side, if Horizon set UK legal precedent than any person or property agent is fully and unequivocally legally liable for the output of any software they use, after the first few are found guilty for things the procedural text generator they used wrote people might decide its not worth the risk.
They are definitely to simple to represent the entirety of an concepts meaning on their own. Yep, I don’t believe it’s likely that such an incrediblely intricate thing as a nuron, much less the idea of conceptual meaning, can be replicated by a high school math problem. Maybe they could be a part, but your off by about a half a dozen order of magnitude at least from where we are now with love being a matrix with a few hundred numbers in it.
No part of a human or animal brain operates on subtracting tables of cleanly defined numbers from each other so I think it’s pretty safe to say that no matrix calculation is done on a handful of numbers as part of much less as our sole means of understanding concepts or objects.
I don’t know exactly how one could tell true understanding from minicry, far smarter and more well researched people than me have debated that for decades, i’m just pretty sure what we think an kindness is boils down to something a bit more complex than a high school math problem discribing a word cloud.
Generally the term Markov chain is used to discribe a model with a few dozen weights, while the large in large language model refers to having millions or billions of weights, but the fundamental principle of operation is exactly the same, they just differ in scale.
Word Embeddings are when you associate a mathematical vector to the word as a way of grouping similar words are weighted together, I don’t think that anyone would argue that the general public can even solve a mathematical matrix, much less that they can only comprehend a stool based on going down a row in a matrix to get the mathematical similarity between a stool, a chair, a bench, a floor, and a cat.
Subtracting vectors from each other can give you a lot of things, but not the actual meaning of the concept represented by a word.
To note the obvious, an large language model is by definition at its core a mathematical formula and a massive collection of values from zero to one which when combined give a weighted average of the percentage that word B follows word A crossed with another weighted average word cloud given as the input ‘context’.
A nuron in machine learning terms is a matrix (ie table) of numbers between zero and 1 by contrast a single human nuron is a biomechanical machine with literally hundreds of trillions of moving parts that darfs any machine humanity has ever built in terms of complexity. This is just a single one of the 86 billion nurons in an average human brain.
LLM’s and organic brains are completely different and in both design, complexity, and function, and to treat them as closely related much less synonymous betrays a complete lack of understanding of how one or both of them fundamentally functions.
We do not teach a kindergartner how to write by having them read for thousands of years until they recognize the exact mathematical odds that string of letters B comes after string A, and is followed by string C x percent of the time. Indeed humans don’t naturally compose sentences one word at a time starting from the beginning, instead staring with the key concepts they wish to express and then filling in the phrasing and grammar.
We also would not expect that increasing from hundreds of years of reading text to thousands would improve things, and the fact that this is the primary way we’ve seen progress in LLMs in the last half decade is yet another example of why animal learning and a word cloud are very different things.
For us a word actually correlates to a concept of what that word represents. They might make mistakes and missunderstand what concept a given word maps to in a given language, but we do generally expect it to correlate to something. To us a chair is a object made to sit down on, and not just the string of letters that comes after the word the in .0021798 percent of cases weighted against the .0092814 percent of cases related to the collection of strings that are being used as the ‘context’.
Do I believe there is something intrinsically impossible for a mathematical program to replicate about human thought, probably not. But this this not that, and is nowhere close to that on a fundamental level. It’s comparing apples to airplanes and saying that soon this apple will inevitably take anyone it touches to Paris because their both objects you can touch.
Like say, treating a program that shows you the next most likely word to follow the previous one on the internet like it is capable of understanding a sentence beyond this is the most likely string of words to follow the given input on the internet. Boy it sure is a good thing no one would ever do something so brainless as that in the current wave of hype.
It’s also definitely becuse autocompletes have made massive progress recently, and not just because we’ve fed simpler and simpler transformers more and more data to the point we’ve run out of new text on the internet to feed them. We definitely shouldn’t expect that the field as a whole should be valued what it was say back in 2018, when there were about the same number of practical uses and the foucus was on better programs instead of just throwing more training data at it and calling that progress that will continue to grow rapidly even though the amount of said data is very much finite.
Except when it comes to LLM, the fact that the technology fundamentally operates by probabilisticly stringing together the next most likely word to appear in the sentence based on the frequency said words appeared in the training data is a fundamental limitation of the technology.
So long as a model has no regard for the actual you know, meaning of the word, it definitionally cannot create a truly meaningful sentence. Instead, in order to get a coherent output the system must be fed training data that closely mirrors the context, this is why groups like OpenAi have been met with so much success by simplifying the algorithm, but progressively scrapping more and more of the internet into said systems.
I would argue that a similar inherent technological limitation also applies to image generation, and until a generative model can both model a four dimensional space and conceptually understand everything it has created in that space a generated image can only be as meaningful as the parts of the work the tens of thousands of people who do those things effortlessly it has regurgitated.
This is not required to create images that can pass as human made, but it is required to create ones that are truely meaningful on their own merits and not just the merits of the material it was created from, and nothing I have seen said by experts in the field indicates that we have found even a theoretical pathway to get there from here, much less that we are inevitably progressing on that path.
Mathematical models will almost certainly get closer to mimicking the desired parts of the data they were trained on with further instruction, but it is important to understand that is not a pathway to any actual conceptual understanding of the subject.
At first glance I thought it was reuseing the coal plants turbines, but looking though the article the only connection I can find is that it’s located several miles away and the only connection is that it plans to hire a hundred or so people from the coal plant it’s replacing and that Wyoming’s powder river basin is nearby and its associated highly automated low sulfur coal mines are in the vauge area.
All this to say, yes it has practically nothing to do with coal.
If it makes you feel any better, modern climate and economic studies have shown that even a full scale nuclear war involving every nuclear power at the height of the Cold War and when nuclear stockpiles were far larger than today we still wouldn’t have come very close to actually killing off all the humans on earth, with the vast majority of the casualties being owed to famine in regions that were/are heavily dependent on western fertilizer. Indeed entire nations in the southern hemisphere tend to get through such senecios without much of an direct effect from world war three.
Mostly this change from earlier predictions came from being able rule out the theory of a nuclear winter as climate modeling became more accurate and we could be sure that the secondary fires from such a war could not carry ash into the upper atmosphere in significant quantities, which was practically shown when a climate change fueled wildfire in Australia got so large that it should have been able to carry the ash into the upper atmosphere under nuclear winter theory but none was observed, validating modern climate models.
Also, dispite what some less scrupulous journalists trying to drum up clicks have posted on the Ukraine War, the Russian government itself hasn’t really made any major signaling moves with regards to bringing nukes into the conflict, and indeed has maintained and repeatedly reiterated Putin’s 2010s no first use policy when asked.
Don’t get me wrong, this is not the result of some greater Russian morals or whatever, but just a consequence of the inherent risk that such posturing could lead to nuclear escalation and breaking the nuclear taboo or even just other nations actually believing they plan to, and such scenarios end very badly for Russia in general and Putin in particular.
It’s also a lot warmer on cold days to have several layers of maxi skirt. I don’t understand how anyone could live without it.
Lower resolution and they operate in different bands of light. Nancy’s main thing is that it has about equal detail to Hubble, but a lot wider field of view. The main mission is to map a lot more of the night sky at decent resolution rather than to get better images of things we’ve already seen.
Then why in the world would he not just say so and just drive or walk to the train between DC and New York? He’s a government official, but not really one notable enough to need a security escort to go to the grocery store.
That doesn’t really address why he uses a source that says that nuclear is absurdly cheap compared to anything else if he also believes that their isn’t any demand to scale up production.
And yes, renewable priceing often does include the cost of the batteries needed for buffering in the commonly cited category of generation+storage. It is worth noting however that this price is much higher than it would be worth a fully renewable grid. This is because not only does it take time to actually build the renewables, which means given their consistently dropping price that by the end the batteries and panels will be cheaper than current prices, but because long range distribution is far cheaper than the direct storage that figure counts.
While renewables are intermittent at a local level, at the continental scale between solar and wind the output is actually very consistent. It costs money to build more HVDC transmission lines of course, but currently thouse are cheaper than batteries or pumped hydro, although that may change if battery prices drop as low as their manufacturers are boasting they will.
Primary energy demand will decrease as more users more to electricity given that most of that energy demand that is currently done with fossil fuels can only being turned into useful work at 40 to 60 percent efficiency, as compared to the 90 percent efficiency typically found with electrical motors. Not that it matters at all, as the only impact primary demand has at all on the cost of sources of electricity generation(the thing we are debating) is that we are going to need to see a large scale up in net electricity generation.
If you have an always on server, you can always run Opnsense as a vm.
Ion and Hall effect thrusters have high efficiencies, but absurdly small thrusts. They work in situations where you can burn for months on end to get the same change in velocity a few minutes worth of a chemical rocket, but would never be able to lift even their own weight off earth.
I also don’t the think that you can even get one to function as deep into the atmosphere as ground level as the would just ark across the potential instead.
You can’t exactly use electricity directly to power a rocket, and fuel represents such a small cost compared to everything else that governments can afford the dedicated production.
Honestly though, the spacecraft themselves are such a tiny emitter compared to things like manufacturing, transport, and electrical generation that they can basically be ignored untill we have basically eliminated thouse emissions.
I am rather skeptical of a source that puts Nuclear at half the cost of gas given it would seem to require that everyone who does the math on private investments decides to half their return for no apparent reason, and it seems odd to claim that spending going up when replacing currently functioning and fossil plants with new solar plants must mean that solar is more expensive long term. The whole point of solar is that it costs a lot up front but very little to maintain, so obviously until all new solar and wind plants are replacing end of life solar and wind plants your going to see an increase in short term cost while building such plants.
It’s also worth noting that solar, battery storage, and wind are new technologies in terms of their current form and are seeing consistent drops in real price as they develop and grow in scale over the last few decades, as compared to far more developed technologies where there is little room for innovation or scale to bring down costs.
I like the bright warning signs, they make a nice whooshing sound as we speed past.
So? OpenAI is to my knowledge open about incorporating conversations into future versions of GPT, and you can often get it to replay training data in full, so your effectively publishing your conversations to the open internet anyway. This feels more like saying that my lemmy comments are stored in plain text on my phone than any sort of security violation.